Recommender systems are time-critical, as the recommendations for a customer must be available without delay to ensure a seamless experience. This is the case with online shopping, for example. When the user adds an item to their shopping cart, further product suggestions should be displayed immediately. Users do not wait for these suggestions. If they don’t come immediately, they move on and the opportunity to sell more products is lost.
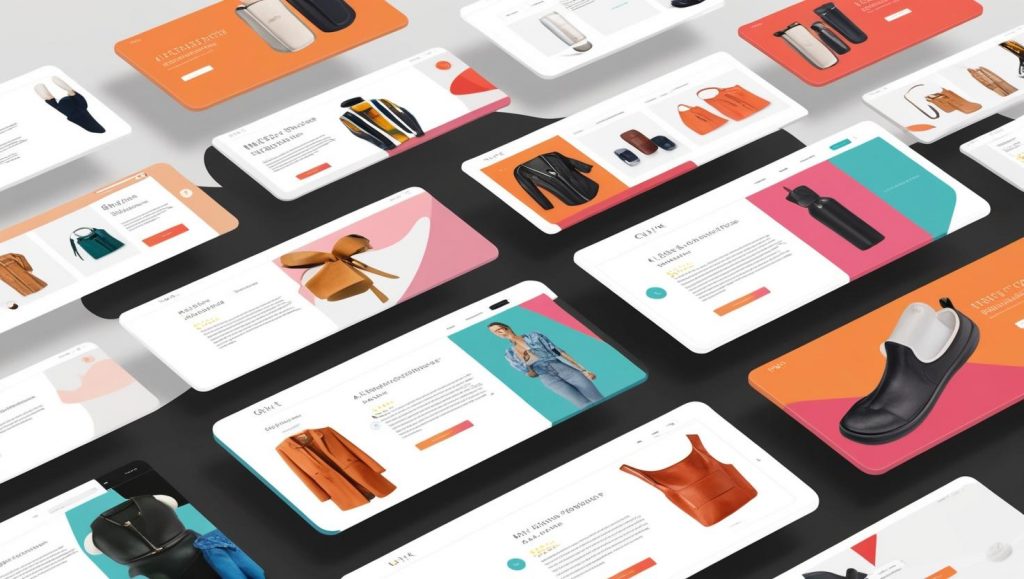
On the other hand, there is usually a high volume of customers that the recommender system is used for. This can lead to high server costs, especially when scaling the service. To prevent costs from exploding, resource-efficient deployment of the underlying models is essential.
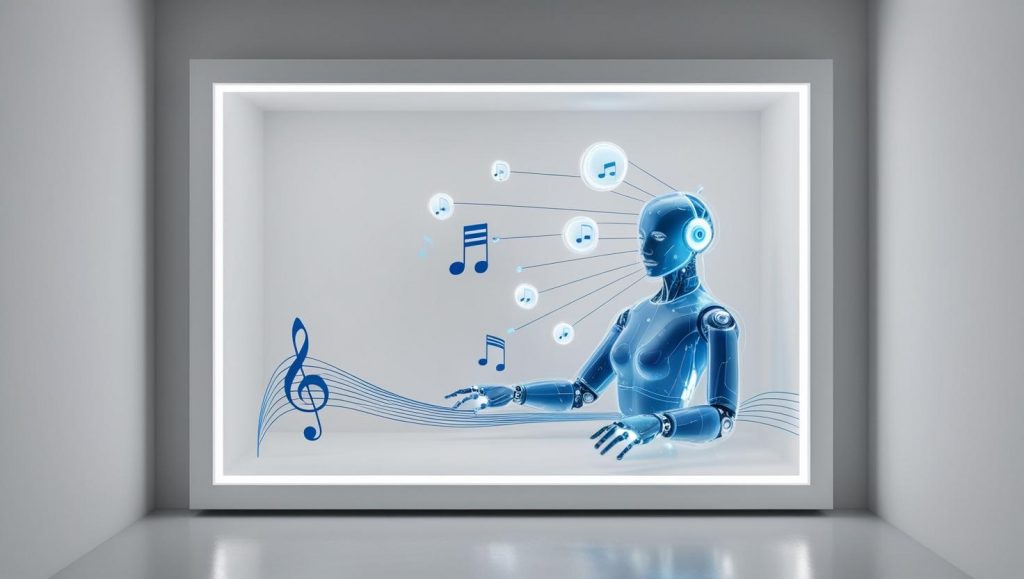
Deployment done right
Deploying artificial intelligence / machine learning models the correct way is easy with our services. Just tell us your requirements and goals, find the right server architecture, and deploy your models seamlessly on-premise or to the cloud. Our all-in-one solution eliminates the hassle, optimizing both your models and deployment configurations to deliver maximum inference speed while minimizing server costs.