Business intelligence is the process of transforming raw data into actionable insights that drive strategic decision-making, and modern business intelligence applications heavily leverage machine learning and artificial intelligence to unlock its full potential. By integrating ML and AI, businesses can go beyond traditional descriptive analytics to predictive and prescriptive insights, enabling them to anticipate trends, optimize operations, and personalize customer experiences. These advanced technologies empower tools to analyze vast datasets, identify patterns, and uncover opportunities that would otherwise remain hidden, providing organizations with a competitive edge in today’s data-driven economy.
Business Intelligence dashboards, for example, serve as dynamic interfaces that provide users with real-time access to critical insights by triggering on-demand data processing tasks. These tasks often include trend analysis, time series forecasting, anomaly detection, customer segmentation, and optimization recommendations, all powered by sophisticated machine learning models. When users interact with such a dashboard – for example by applying filters, drilling down into data, or requesting forecasts – underlying ML models must process the queries and deliver results instantly. Any delay in this process can disrupt workflows, cause frustration, and ultimately lead to poor user experiences. This underscores the importance of deploying these models on high-performance inference servers capable of handling computations with ultra-low latency. Ensuring rapid execution not only enhances productivity but also solidifies trust in the system’s reliability and responsiveness.
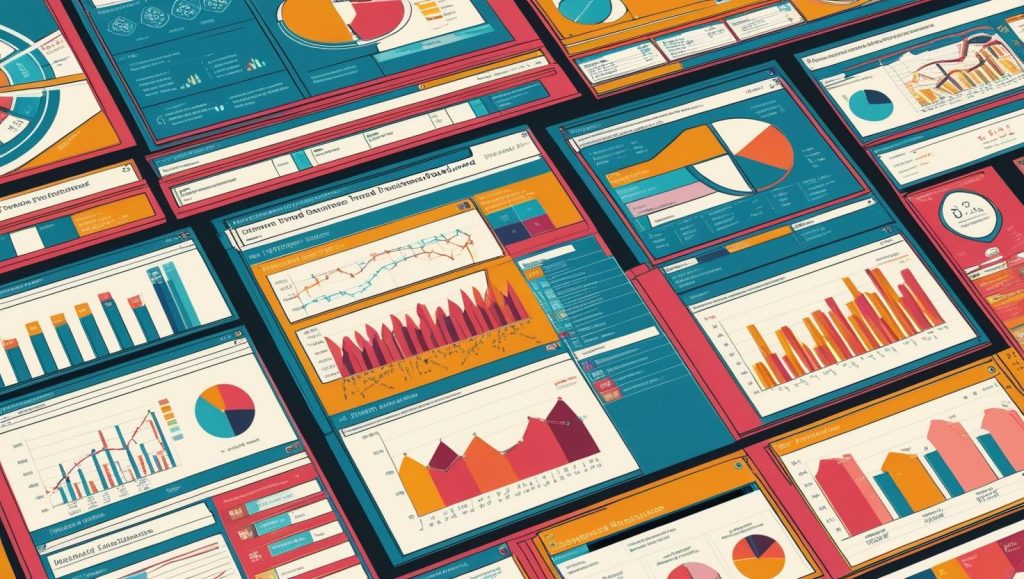
Even if the processing of requests for machine learning models in time is the main focus, the resource requirements for these models must not be neglected. Latency requirements often go hand in hand with high server costs for deployment. This is where we come into play with our tools. On the one hand, we make the models faster so that they can be deployed on-premise or in the cloud. On the other hand, we also focus on minimizing resource requirements and selecting suitable hardware, which results in significantly lower server costs.
Deployment done right
Deploying artificial intelligence / machine learning models the correct way is easy with our tools. Just define your models in our web-app, find the right server architecture, and deploy your models with a single click on-premise or to the cloud. Our all-in-one solution eliminates the hassle, optimizing both your models and deployment configurations to deliver maximum inference speed while minimizing server costs.